Face to Face: Why NIR Facial Recognition Systems Are the Most Secure
Facial recognition and secure ID applications are becoming increasingly common in our digitized world, used by law enforcement, banking, phones, and more. And there are a range of different technologies in use, including thumbprint sensors, photographic image matching, thermal imaging, and near infrared (NIR) sensing. Depending on the use case, each of these technologies can be effective. But when it comes to the personal security of our devices and homes, nothing beats NIR sensing for accuracy.
Identification System Technologies
There are two main types of facial recognition systems: 2D systems that rely on analysis of two-dimensional photographic or video images of a person’s face, and 3D systems that use near-infrared NIR light patterns to detect facial geometry. Recently, some thermal imaging FR technologies have also emerged. Most of the facial recognition (FR) that we hear about in the news is the 2D type. For example, law enforcement can use FR software to analyze photographs of an event and identify people who instigated violence.1 Photographic FR is also used to check faces at airport security and help find missing persons.
However, 2D facial recognition is not always accurate; false identifications occur, variations in lighting and photo angles can confound a match. These systems have even been fooled by analyzing a 2D image of a person’s face rather than the live person. Video matching can improve accuracy, but even these systems can be fooled by today’s “deep fake” videos.
More secure and foolproof than 2D photographic FR is fingerprint identification. “Fingerprint recognition systems work by examining a finger pressed against a smooth surface. The finger’s ridges and valleys are scanned, and a series of distinct points, where ridges and valleys end or meet, are called minutiae. These minutiae are the points the fingerprint recognition system uses for comparison.”2
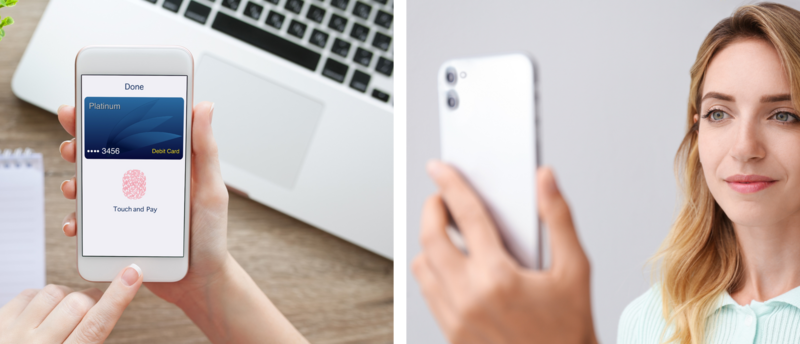
Fingerprint (left) and facial recognition (right) features are common on today’s smartphones.
But savvy criminals can still outsmart fingerprint ID systems with $5 - $15 worth of supplies3 (or, if some movies are to be believed, a piece of scotch tape is all you need—not true). Some security systems combine facial + fingerprint modes to foil hackers. But NIR-based systems still outperform all the other identification methods.
How NIR Identification Works
NIR systems include both facial and retinal scanners that operate in 3D. NIR FR works by casting a pattern of invisible NIR light—from an LED or laser emitter—on a person’s face to measure not only two-dimensional elements such as the distance between our eyes, but also depth, such as the length of our nose. By creating a 3D “map” of the face, NIR FR systems can be much more accurate.
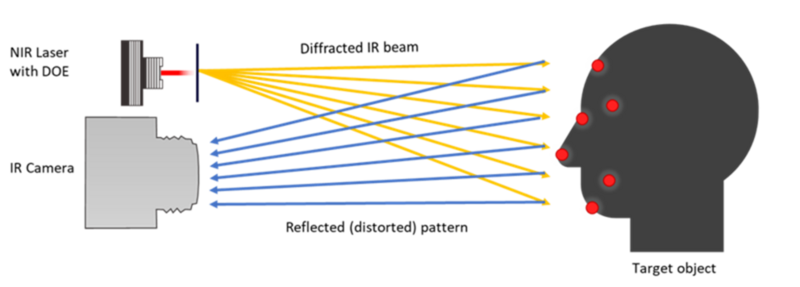
NIR facial recognition systems cast NIR light through a DOE filter towards the face (yellow beams) in a defined pattern and capture the reflection of that pattern with an NIR camera (blue beams), to measure distortion caused by facial contours.
Other advantages of 3D FR technology include:
- Ability to measure faces in low light or darkness
- Ability to correctly identify faces when viewed from different angles
- Secure from “spoofing”—using the photo of a person’s face to try and fool an FR system.
NIR-based retinal scanning is based on the fine capillary network in the back of our eye. “As a person looks into the eyepiece, an invisible beam of low-energy infrared light traces a circular path on the retina at the back of the eye. The blood-filled capillaries absorb more of the infrared light than the surrounding tissue. Because of this, there is a variation in the intensity of the reflection,”4 which is measured by the scanner. Due to its superior accuracy, retinal scanning is used by the U.S. Pentagon among other high-security facilities.
NIR-Based System Parameters
With their superior accuracy, why aren’t NIR-based identification systems being used everywhere? For one, they are a bit more complex and expensive to implement than simply using software algorithms to analyze photos or videos. NIR identification requires a “system-level solution” with multiple hardware components including an emitting source to send out NIR light waves, and a camara/scanning device that receives the reflected light waves so they can be interpreted and matched.
NIR emitters also require careful implementation to protect the user from dangerous levels of infrared wavelength that can cause damage to the human eye with intense or prolonged exposure. It’s important to measure NIR sources—LEDs and lasers (VCSELs)—to ensure they are emitting within a specified wavelength and radiant intensity, and that any light pattern distributions are accurate.
Quality Measurement of NIR Emitters
Manufacturers of FR systems and components need to measure NIR sources for emission uniformity and distribution, spatial positions of pattern elements, radiant flux, and maximum intensity or power—across the entire distribution area. For NIR facial-recognition systems and retinal scanning—and other NIR 3D sensing applications such as gesture control, proximity sensing, eye tracking, and object matching—Radiant offers a solution for NIR light source measurement that is helping companies ensure performance and accuracy: the NIR Intensity Lens and TT-NIRI™ Software.
Learn more about NIR measurement in the webinar, Measuring LEDs and Lasers for Near-IR Sensing Applications. It includes discussion of:
- Near-IR sensing trends for smart devices, AR glasses, and VR headsets
- Considerations for ensuring performance and safety of near-IR LEDs and lasers (VCSELs) used for facial recognition and other 3D sensing applications
- The fastest method for direct angular measurement of NIR intensity, beams, and patterns
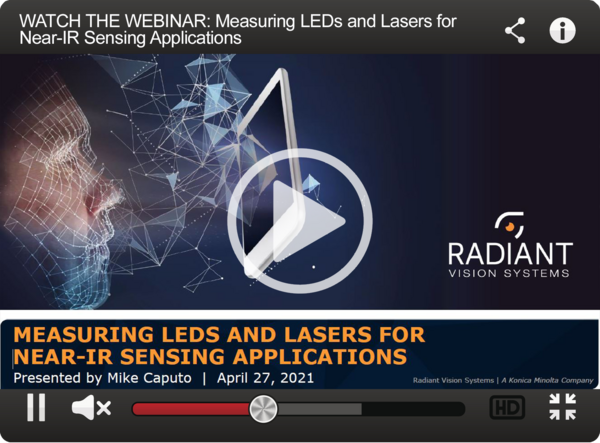
CITATIONS
- Hill, K., “The facial-recognition app Clearview sees a spike in use after Capital attack.” New York Times, January 9, 2020.
- Devi, R.M., et al, “Chapter 5 - Retina biometrics for personal authentication,” in Editor(s): Sarangi, P.P, et al., Cognitive Data Science in Sustainable Computing, Machine Learning for Biometrics. Academic Press, 2022, Pages 87-104, ISBN 9780323852098. https://doi.org/10.1016/B978-0-323-85209-8.00005-5.
- Mott, N., "Hacking Fingerprints is Actually Pretty Easy-and Cheap", PC Magazine, November 22, 2021.
Join Mailing List
Stay up to date on our latest products, blog content, and events.
Join our Mailing List