Facing the Future: New Applications and Trends in Facial Recognition
There are 62 million security cameras in the U.S. alone,1 and roughly 650 million facial recognition (FR) cameras worldwide,2 with millions more FR-capable devices carried around every day in people’s pockets. Facial recognition technology has been around for quite some time, but new advances in 3D sensing, artificial intelligence, and machine learning are expanding its application in ways that may fundamentally change aspects of government, industry, and law enforcement activities.
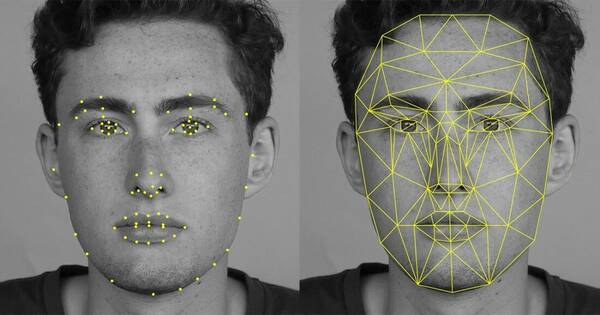
Airports are beginning to use facial recognition to cross-check passengers for international boarding. Image Source.
Faces offer an advantage over other biometric authentication methods such as fingerprints or iris scanning because they do not require active participation by the subject: a camera can capture anyone’s face solely based on proximity.
Facial recognition can be broadly categorized into three types of functions:3
- Basic FR systems simply recognize the presence of a face for purposes such as applying “Animoji” images and Instagram filters. A camera looks for the defining features of a face (a pair of eyes, a nose and a mouth), and algorithms help determine the direction and movement of the face, for example whether the mouth is open or closed. There is no measurement of unique facial features or individual identification.
- Face Identification. These applications work by first storing an image of a person’s face by measuring the unique features that will identify it. To access a device or facility, the person must present their face again, and a facial recognition system measures their features and confirms their identity against the stored face.
- Face Searches. Some systems are used to identify unknown faces for security, advertising, or law enforcement purposes. They operate similarly to a face identification function, but instead of matching one person to one stored reference face, algorithms search an extensive database of faces to find a match.
2D vs. 3D Facial Recognition Systems
Most FR software in use today relies on two-dimensional (2D) technology, namely, photographs or video images. These images are easy to obtain, for example, by using footage from a security camera or a person’s Facebook profile picture.
However, using 2D images for FR also has some drawbacks. Because photographs are flat, algorithms can measure certain features—such as the distance between a person’s eyes—but cannot measure depth or dimensions such as the length of a nose or a prominent chin. Additionally, 2D recognition systems are dependent on the visible light spectrum to acquire an image. If ambient lighting conditions are dark or murky, it can impede recognition accuracy.
The more recent emergence of three-dimensional (3D) sensing systems based on invisible near-infrared (NIR) light offers increased detail and eliminates a reliance on ambient light. NIR-based facial recognition systems work by casting a pattern of tiny dots (created by shining near-infrared laser light through a filter, called a diffractive optical element or DOE) onto a person’s face. An NIR camera captures the reflection of those dots, using alterations in the reflected pattern and/or time of return to create a 3D “map” of the person’s face.
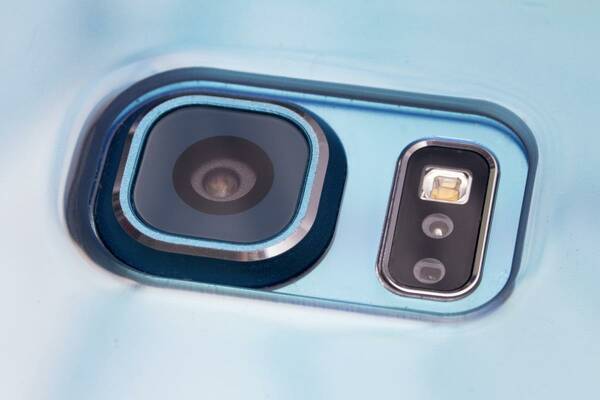
Close up of near-infrared light emitter on a smart phone.
Within the categories of 2D and 3D facial recognition, there are, of course, many variations of technology implementation including hybrid (multi-modal) systems.
Emerging Facial Recognition Use Cases
Many of the current and most obvious uses of FR technology have been in the realms of security (e.g., controlling access to a device or facility to only authorized individuals), public safety, and law enforcement (e.g., FR was used to identify a shooting suspect in Washington, D.C.)
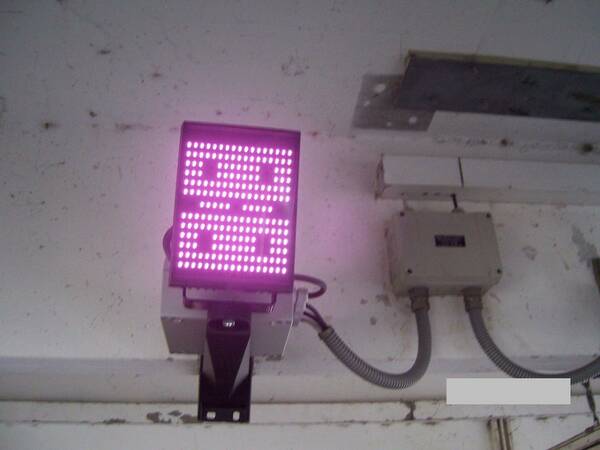
Camera system in a Swiss parking garage that performs facial recognition; identifies vehicle make, model, and color; and reads the license plate.
When biometric data is optimized and combed with artificial intelligence or machine learning capabilities, the possible applications are vast. A few examples:
- Automaker Subaru is the first to introduce facial recognition into it's latest Forrester model. The sytem combines an infrared LED and a camera to monitor the driver for signs of inattention or sleepiness, warning them if needed. The system recognizes up to five individual drivers, so settings and preferences can be automatically adjusted for each person.
- Hotels are considering using facial recognition systems to identify and even interact with guests, with 72% of hotel operators in one survey expecting to deploy such technology in the next four years.1 When guest belong to loyalty programs, facial recognition can enable new levels of concierge service and perks.
- In healthcare, deep learning, and face analysis have already made it possible to:
- “Track a patient's use of medication more accurately
- Detect genetic diseases such as DiGeorge syndrome with a success rate of 96.6%
- Support pain management procedures.”4
- For health care providers in assisted living or memory care facilities, facial recognition can offer an additional layer of patient protection, for example, if a patient wanders off the property.
- Retail is another hot area for FR applications with stores like Amazon Go and Saks Fifth Avenue already testing ways to link customers to their online profiles, track their activity in store, and more.
- Taking retail integration to the next level, both fried chicken chain KFC and Chinese retail & tech giant Alibaba have been testing face recognition payment solutions.
- Beyond just facial recognition, the field of computer emotion recognition is also growing, using analysis of geometric composition and appearance of facial features plus parameters extracted from transformed images such as eigenfaces, dynamic models, and 3D models.4
The potential for increased safety, security, and convenience from advanced facial recognition systems is significant. The current 3D FR systems have a 99.44% successful acquisition rate of accurate facial images in under five seconds.5 However, there are some privacy and other concerns about the expanding use and potential misuse of facial recognition technologies, and risks if systems make a false identification or are hacked. While these considerations are outside the scope of this post, they are discussed by BBC News and by Phys.org.
Ensuring the Quality of NIR Facial Recognition Devices
At Radiant, our work in facial recognition has been primarily focused on the performance of device security for products such as smartphones and tablets, automobiles, and other consumer-focused applications that use NIR-based 3D sensing technologies.
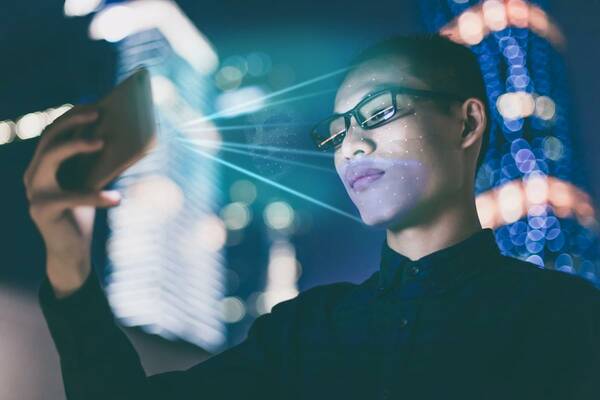
Simulation of a facial recognition system projecting an invisible NIR dot pattern onto a user’s face to unlock his phone.
With the rapid adoption of 3D NIR sensing systems comes a growing demand for effective methods to measure the accuracy of NIR emitters. While 3D NIR technology provides more accurate facial recognition than previous 2D (photographic) methods, NIR systems can still be subject to performance issues. What if the NIR emissions are inaccurate in spatial scope, position, or intensity? What happens when low-output or poorly placed emissions are interpreted by the sensing device?
There are also safety considerations working with NIR wavelengths. They are invisible to humans, so do not trigger an “aversion response” (blinking or looking away from bright light). Yet NIR wavelengths can enter the eye and—with too much power (too much irradiation per area)—can cause damage to the retina or cornea. For safety reasons, facial recognition and eye detection systems that emit light in the NIR range must be carefully designed and tested to ensure they are emitting at correct levels.
For device quality, accuracy, and performance, manufacturers employ measurement methods to test the design and manufacture of NIR light sources (primarily near-IR LEDs and VCSEL lasers ). Ideally, a measurement system captures a variety of different characteristics such as emission uniformity, maximum power or intensity, radiant flux, emission distribution, or spatial position—and it measures these parameters across the entire distribution area.
The Radiant Vision Systems Near-Infrared (NIR) Intensity Lens system is an integrated camera/lens solution that measures the angular distribution and radiant intensity of 850 or 940 nm NIR emitters. The system uses Fourier optics to capture a full cone of emission data in a single measurement up to ±70 degrees inclination and 360 degrees in azimuth, giving you extremely fast, accurate results ideal for in-line quality control.
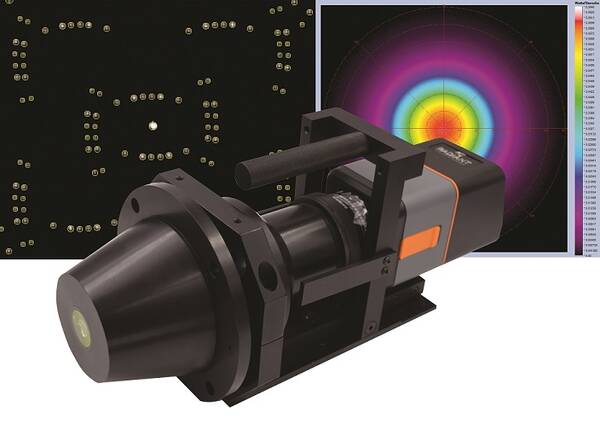
Radiant’s Near Infrared (Near IR) Intensity Lens solution, including specialty lens, integrated ProMetric® Y16 Imaging Radiometer, and TT-NIRI™ Software.
Manufacturers of 3D sensing technology can apply the NIR Intensity Lens solution for angular measurement of NIR LEDs, lasers, and structured light patterns produced by Diffractive Optical Elements (DOE). The lens is integrated with a Radiant Vision Systems ProMetric® Y16 Imaging Radiometer, and features ProMetric® or TrueTest™ Software for intuitive system setup and customizable automated measurement sequences. Additional tests specific to NIR emission measurement are available in the TT-NIRI™ software module.
To learn more about ensuring the accuracy of NIR emissions, read the white paper: “Measuring Near-Infrared (NIR) Light Sources for Effective 3D Facial Recognition.
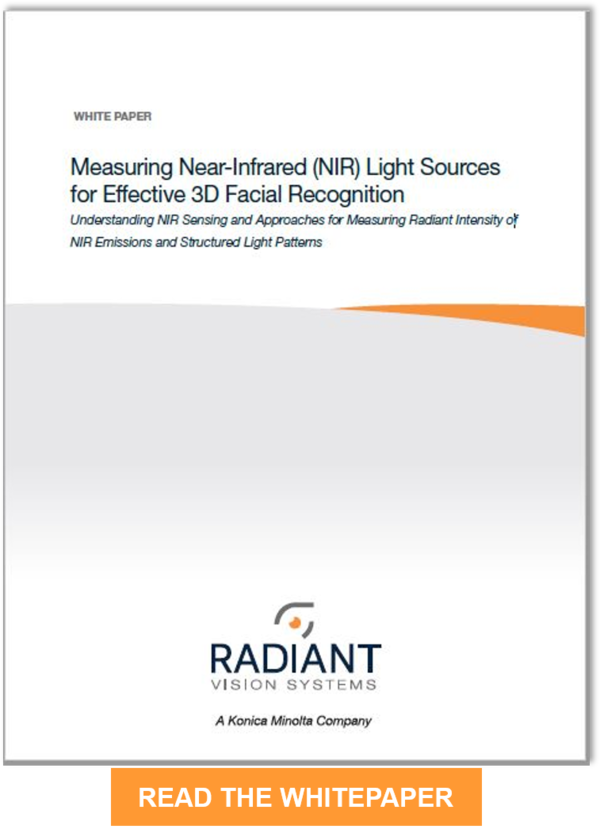
CITATIONS
- Xie, M., “The Future of Biiometric Facial Recognition”, Forbes, September 19, 2008. LINK
- Heinzman, A., “How Does Facial Recognition Work?”, How-To-Geek, July 11, 2019. LINK
- Kharkovyna, O., “Facial Recognition And AI: Latest Developments And Future Directions”, Medium, February 1, 2019.
- “Facial recognition; top 7 trends (tech, vendors, markets, use cases and latest news)”, published on Gemalto.com, retrieved August 29, 2019. LINK
- Results from the 2018 Biometric Technology Rally sponsored by the Department of Homeland Security Science and Technology Directorate, as reported by Gemalto.com, 2019. LINK
Join Mailing List
Stay up to date on our latest products, blog content, and events.
Join our Mailing List